Unlocking Insights: Validation of Results in Statistical Models and MU Identification Accuracy
On January 29th, 2024, Professor Aleš Holobar and Nina Murks hosted a webinar titled "Validation of Results: Statistical Models and MU Identification Accuracy," delving into the intricate realms of motor unit (MU) identification accuracy, regression analysis with linear mixed models, and Bayesian linear regression models.
The webinar commenced with a comprehensive examination of MU identification accuracy from High-Density Electromyography (HDEMG) signals. Participants gained insights into MU spike trains, filters, discharge patterns, and rates, setting the stage for a discourse on information extraction pipelines and BBS-based MU firings identification.
One of the webinar's highlights was the exploration of dataset simulation, shedding light on the process of synthesizing EMG signals and measuring experimental surface EMG. Attendees also delved into the challenges posed by MU merging and the deceptive nature of Pulse-to-Noise Ratio (PNR), gaining a deeper understanding of the intricacies involved in accurate data interpretation.
As the session progressed, participants were immersed in the world of regression analysis, with practical demonstrations of coding in MATLAB and R. The importance of model assumptions and the visual inspection of data were emphasized, underscoring the significance of experienced interpretation in ensuring reliable results.
A pivotal moment in the webinar was the exploration of different models of linear regression, including Linear Mixed Effect and Bayesian regression approaches. Attendees gained insights into evaluating model performance using metrics such as AIC and BIC, empowering them with tools to assess the efficacy of their analyses effectively.
The webinar concluded with a reflection on the diverse approaches to data analysis, highlighting the importance of considering various programming languages and packages to support linear regression models. Attendees gained insights into different schools of thought, including Linear Mixed Effect and Bayesian Linear Regression, and the implications of different data models on analysis outcomes.
In summary, the webinar provided an overview of validation techniques in statistical models and MU identification accuracy, offering attendees a wealth of knowledge and practical insights to enhance their research endeavors.
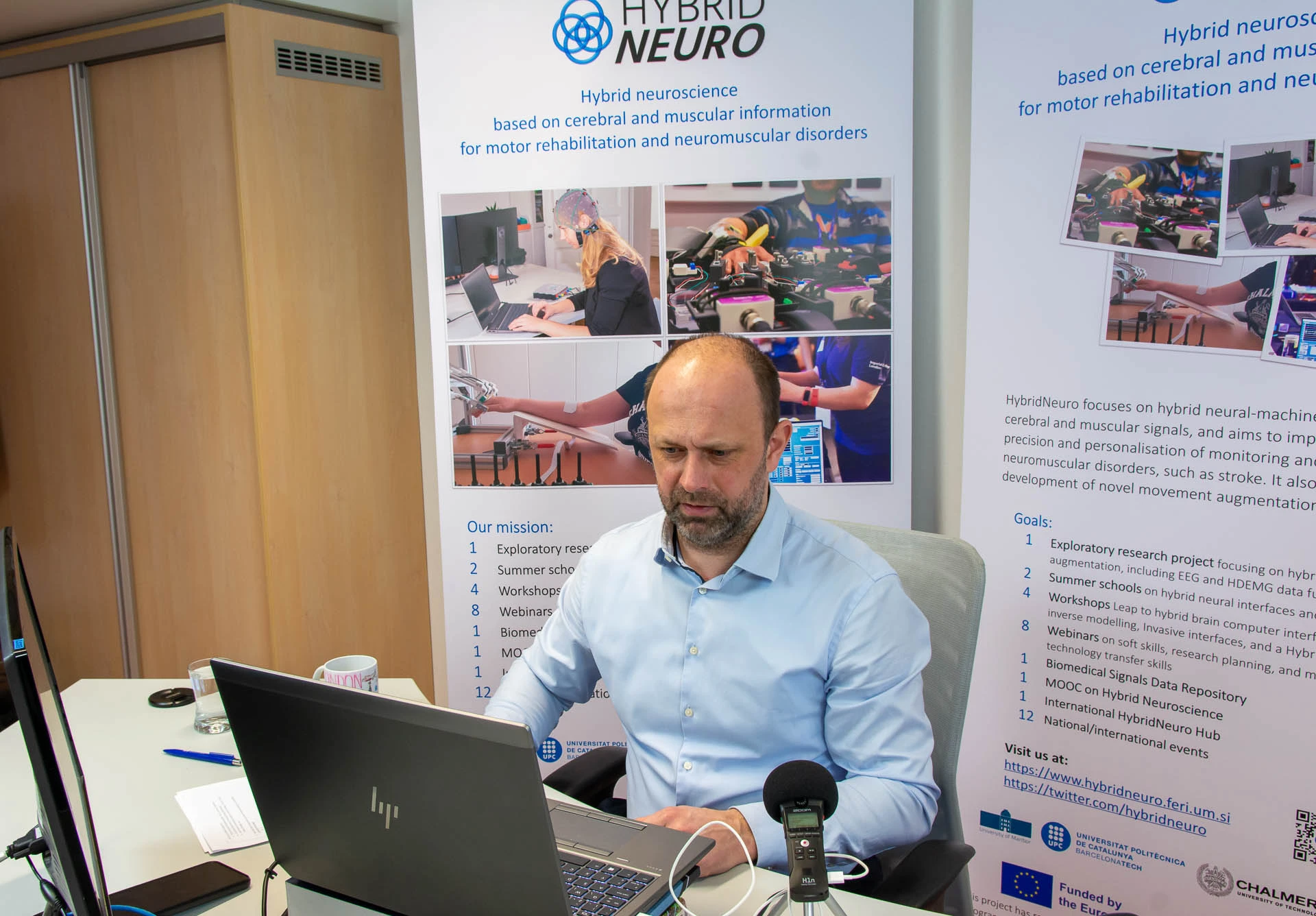